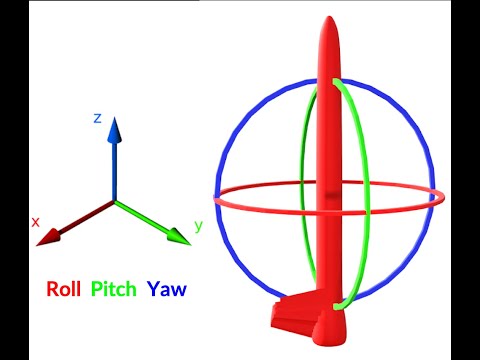
Self-landing rockets have been theorized over the past century but until 2015 no one had ever managed to bring a rocket into outer space and successfully complete a stable re-entry with a thrust-landing. Reinforcement Learning (RL) is a technique that without a model has promising ability to understand the best action to be taken in a given state. RL allows for instantaneous decision making, whereas the most common approach of Model Predictive Control (MPC) requires to simulate steps ahead. We are hopeful that the results from
RL have the ability to create a robust policy that can drastically outperform MPC’s with regard to its computation requirements, as well as effectively approximate the actions within an acceptable bound.
The entire field has been overwhelmingly developed under the influence of Control Theory, which involves solving approximated physical dynamics of the real world at each decision step of the system. This approach is known as the receding horizon: through simulated steps. Long term predictions are unfeasible because incremental errors accumulate during each simulation step, thus decisions must be made with a limited finite horizon. SpaceX uses a Control Theory, with an on-board computer leveraging a receding horizon to predict its state by generating an open-loop state and control trajectories. Other individuals have analyzed the performance comparison between MPC and RL methods, such as R. Ferrante [1], but in most cases the analysis is approached in a 2-dimensional environment, with the aid of lateral thrusters.
Our approach to solve rocket landing leverages the OpenRocket simulator as an environment, the best open-source model rocket simulator [2].
By focusing on the analysis of landing the rocket in the 1-dimensional case (only allowing motion along the Z-axis) and using Monte Carlo with optimistic initial values, we have shown that the task of landing the rocket is relatively simple even considering the relatively large state space aggregation. We are continuing the research by studying an effective way to complete the learning with the coupled MDP, in which the stabilizer is able to learn in tandem with the lander. Our next step is to increase the simulated motor accuracy by leveraging the thrust curve and not only the thrust percentage. Additionally, we are interested in incorporating coordinate location to the state definition, allowing for fully guided trajectory landing.
References:
[1] R. Ferrante. A robust control approach for rocket landing. Master’s thesis, University of Edinburgh, 2017.
[2] S. Niskanen. Development of an open source model rocket simulation software. Master’s thesis, Helsinki University of Technology, 2009. simulation-thesis-v20090520/Development_of_an_Open_Source_model_rocket_simulation-thesis-v20090520.pdf
0 Comments